
Video Web:A network of a large number of wireless/wired video network.
3D object recognition from range images
A new integrated local surface descriptor is developed for efficient surface representation and surface matching.
Super-resolution of facial images locally in video
|
We propose a new method for enhancing the resolution of low-resolution
facial image by handling the facial image non-uniformly. We segment facial image into different
regions corresponding to different motion models and estimate the motions non-uniformly of tracked
regions in the consecutive frames. The experimental results provide a proof of the concept for our
method and show that our method gives better results than handling the face uniformly. |
(a)
(b)
(c)
(d)
(a) LR Image
(b) Bicubic interpolated image
(c) Uniformly reconstructed image
(d) Non-uniformly reconstructed image
|
Happy Sad Surprise
Disgust
Facial Expression recognition
Unlike current research on facial expression recognition that generally selects visually meaningful feature by hands, our
learning method can discover the features automatically in a genetic programming-based approach that uses Gabor wavelet representation for primitive features and
linear/nonlinear operators to synthesize new features.
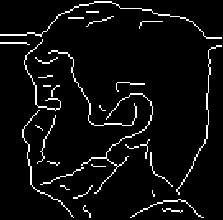
Face Profile Recognition from a Video Resolution enhancement algorithm is first employed to construct a high-resolution face profile image from multiple low-resolution face profile images that are extracted from video.
A curvature-based matching approach is presented for face profile recognition. A dynamic time warping method is used to match the face profile portion from nasion to throat.
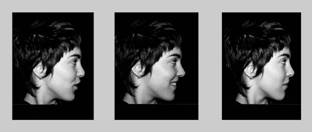
Gait Recognition
Fusion of side face and gait for Human Recognition at a Distance in Video
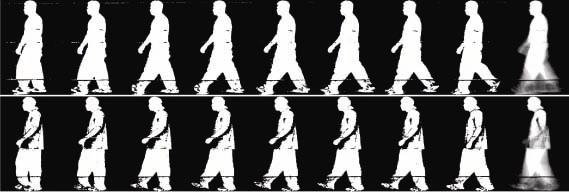
Anomalous human activity detection in video sequences Based on gait energy image (GEI) and co-evolutionary genetic programming (CGP) classify human activities.
Example GEIs of the walking person carrying a briefcase (above) and without briefcase (below)
|
|
|
|
|
|
3D Modeling of Walking Humans
Representative poses of walking subjects are captured. We fit 3D human body model to the captured 3D body data.
|
|
|
|
|
|
|
|
The model of the human body is based on a kinematic tree consisting of 12 segments. Each body segment is approximated by a 3D tapered cylinder.
|
|
|
|
|
Linear interpolation allows gait reconstruction. The body model is walking from right to left at a constant speed.
|
Audio-video Sensor Fusion for Walking Human Detection and Tracking Moving object detection plays an important role in automated surveillance systems. We compare two approaches, the time-delay neural network and Bayesian network, for detecting moving humans using multi-modal measurements
Audio-video S ensor Fusion for Walking Human Detection and Tracking
Example of detection results with the walking person marked by the white circles and bounding boxes. The other one is making local motions, such as waving hands and turning his body, to “fool” the system.
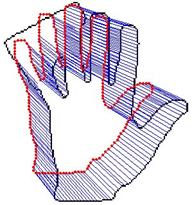
Non-rigid 2D shape registration We develop a to-local procedure for aligning non-rigid shapes is presented. The global similarity transformation is obtained based on the corresponding pairs found by matching shape context descriptors. The local deformation is performed within an optimization formulation, in which the bending energy of thin plate spline transformation is incorporated as a regularization term to keep the structure of the model shape preserved under the shape deformation.
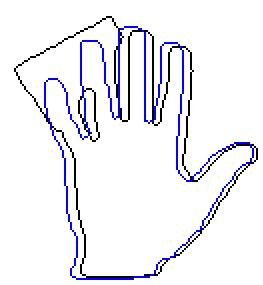
Ear helix/anti-helix representation The 3D coordinates of the ear helix and anti-helix parts are obtained from the ear detection procedure.
Fusion of color and range images and global-to-local registration A single reference 3D ear shape model is used to accurately locate the ear helix and the anti-helix parts in registered 2D color and 3D range images.
Ear shape model based registration The ear shape model is represented by a set of discrete 3D vertices corresponding to ear helix and anti-helix parts.
Template matching based detection The model template is represented by an averaged histogram of shape index calculated from the principal curvatures.
Local surface patch representation
LSP, a local surface descriptor, is characterized by a centroid, a local surface type and a 2D histogram. The 2D histogram shows the frequency of occurrence of shape index values vs. the angles between the normal of reference feature point and that of its neighbors. It is found to be more efficient then spin image representation
Binomial prediction model for fingerprint recognition binomial model to predict both the fingerprint verification and identification performance. The match and non-match scores are computed, using the number of corresponding triangles as the match metric, between the query and gallery fingerprints.
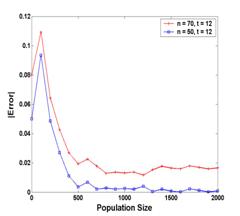
Binomial prediction model Experimental results for the binomial prediction model: (a) Distribution of the match score. (b) Distribution of the non-match score. (c) Absolute error between the experimental and predicted verification performance. (d) Absolute error between the experimental and predicted identification performance.
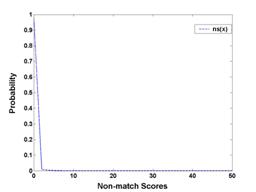
Uncertainty Handling in Spatial Databases Unlike the traditional fuzzy approaches in relational databases, in our work a probability-based method to modal and index uncertain spatial data is proposed.
Comparison of Indices for Uncertain Spatial Databases By performing a comprehensive comparison among OGMH, UR1, UR2 and a standard R-tree on U.S. Census Bureau TIGER/Line® Southern California landmark point dataset, it is found that UR1 is the best for certain queries. However, only OGMH is applicable for uncertain queries.
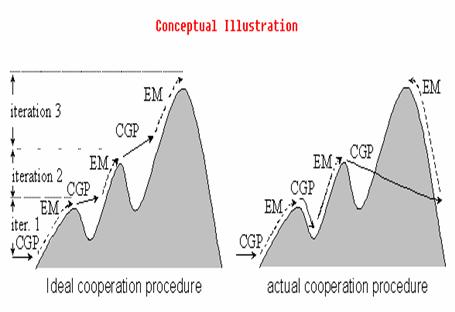
Co evolutionary feature synthesized EM algorithm CFS EM As a commonly used unsupervised learning algorithm in Content-Based Image Retrieval (CBIR), Expectation Maximization (EM) algorithm has several limitations, including the curse of dimensionality and the convergence at a local maximum.
Comparison of CFS-EM and Other Classifiers in Image Retrieval CFS-EM is especially suitable for image retrieval because the images can be searched in the synthesized low-dimensional feature space, while a kernel-based method has to make classification computation in the original high-dimensional space.
Physical Models for Moving Shadow and Object Detection in Video Current moving object detection systems typically detect shadows cast by the moving object as part of the moving object. Unlike previous work, we have developed an approach that does not rely on any geometrical assumptions such as camera location, and ground surface/object geometry.
Two examples from two different scenes with moving vehicles where shadow of vehicles either follows or precedes them. The scene includes both asphalt with different texture, and concrete.
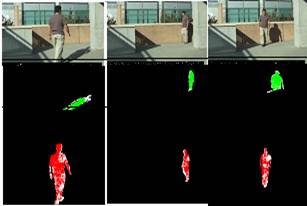
Shadows are cast on different vertical and horizontal surfaces
This represents different types of background surfaces including vertical, horizontal, textured, uniform, brick and concrete. The detection algorithm performed consistently for all the geometry and surface types present in this example.
Physical Models for Object Detection in Video This represents an inclined and curved grass surface. As the subject and its shadow move closer to the camera the detection improves. This is also an example of a surface that exhibits highly saturated color and secularities due to the surface type of grass and angles of incidence. This is a challenging test since we do not account for secularities that introduce noise, which affects the estimation of Cb.
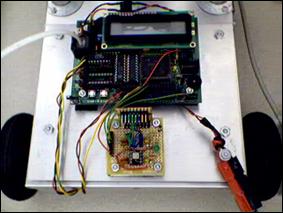 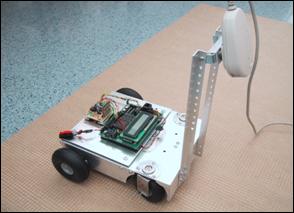
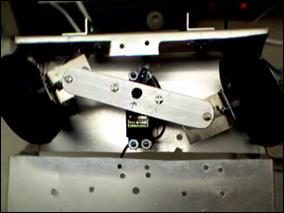
Learning-Integrated Perception Based Speed Control
-- Design a robot system that can learn to drive over a given terrain at an appropriate speed using vision and surface roughness.
-- Build a robot platform for real time testing.
3D Modeling of vehicles
|
Color video data of moving object provides multiple 2D views of the same
object with statistical spatio-temporal relations between appearing and
disappearing features of the object. Modeling of these spatio-temporal
feature dynamics can lead to building of the 3D model of the object from
2D color-video. We are working in this direction, with special focus on
rigid objects at first (later can be extended for flexible objects), with
unsupervised incremental learning of the 3D model of the object.
Ghosh--VehicleModeling
|
Unsupervised Learning for Incremental 3-D Modeling
|
For learning based incremental 3D model-building of rigid objects from
2D video of the object in motion, we use a novel TEMPLATE-LIBRARY for 2D-to-3D
mapping of features and adopt a incremental dynamic clustering technique.
Template concept counters fore-shortening from projection. We also developed a
novel RELIABILITY-based hierarchical structural performance measure to evaluate
and (incrementally) learn at the same time.
AAAIworkshop'05 final paper
|
A Psychological Adaptive Model for Video Analysis
|
One crucial question in video-indexing so far unaddressed is
"How many keyframes to be selected to represent a video?" We
develop a psychological adaptive model to estimate this number,
online, based on "Human Visual Perception and Attention Theory"
and information-content of Commercial Movie-Clips with varied complexity.
The scheme, incorporated with one automatic optimal key-frame selection method
and multiple frame-based features, has been compared to general ad-hoc key-framing
methods, by "Effectiveness score", a novel performance measure developed by us.
Ghosh-KeyFrame
|
Face Tracking and Video
|